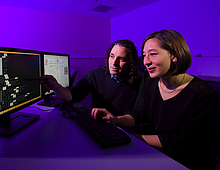
Three faculty in the University of Maryland Institute for Advanced Computer Studies (UMIACS) have received AWS Machine Learning Research Awards for projects that involve the use of artificial intelligence in mental health research, making neural machine translation more trustworthy, and exploring new concepts in deep learning.
Philip Resnik, Marine Carpuat (left, right in photo) and Larry Davis will serve as principal investigators on the projects. Resnik and Carpuat are part of the Computational Linguistics and Information Processing (CLIP) Laboratory, while Davis is director of the Center for Automation Research (CfAR).
The Amazon program funds eligible universities, faculty, doctoral students and postdocs that are conducting novel research in machine learning. Its goal is to enable research that accelerates the development of innovative algorithms, publications, and source code across a wide variety of machine learning applications and focus areas.
The program also allocates credits to use Amazon Web Services (AWS). These credits grant researchers and their students access to Amazon’s distributed cloud offerings, where resources like large-scale storage and computation capabilities that will scale-on-demand are available.
Resnik, a professor of linguistics and director of the CLIP Lab, is working with Han-Chin Shing, a third-year computer science doctoral student. Their project, “Tackling the AI Mental Health Data Crisis,” is designed around the idea that the greatest progress in AI happens when a whole research community can work on shared problems and common datasets.
Resnik says that such datasets are few and far between in research involving mental health. At the same time, he adds, people are spending more time on social media, and computational researchers are developing new and increasingly promising methods for assessing and monitoring mental health conditions using behavioral and language signals in social media data.
Resnik and Shing want to build-out a computational framework for the voluntary donation of social media and questionnaire data, to recruit data donors at scale, and to construct a secure, ethically-governed virtual data enclave on AWS that provides AI researchers with both access to the data and the compute cycles they need to make progress.
The end goal is a practical, sustainable solution to the crisis-level lack of data for AI research in mental health, Resnik says.
Carpuat, an assistant professor of computer science, says her project is focused on making neural machine translation more trustworthy.
She notes that deep learning has recently brought machine translation into a higher performance regime, which makes it possible to deploy translation systems in new challenging use cases. However, it also creates new failure modes: when errors are less obvious, users can be misled into trusting incorrect translations.
Carpuat’s project looks to make neural machine translation more reliable by automatically detecting potentially misleading translations, and by improving neural machine translation architectures to avoid this issue in the first place.
Carpuat says her Amazon-funded research will build on the CLIP Lab’s prior work involving learning representations of word and sentence meaning.
Davis, a Distinguished University Professor of computer science, says his award will be shared with fellow UMIACS faculty members Rama Chellappa (electrical and computer engineering), David Jacobs (computer science), Tom Goldstein (computer science) and Furong Huang (computer science).
The team will investigate a set of fundamental research questions on the foundations and applications of deep learning. They hope to answer questions such as how to make deep learning interpretable to humans.
Davis says a critical barrier to the creation of fully autonomous and robust systems is the lack of interpretability and reliability of deep classifiers. Unfortunately, the power gained from using a neural network is often negated by the lack of explainability and interpretability of such models.
Another question the team will consider is how to effectively and economically train deep networks on new tasks and domains. Davis says one of the classic problems in pattern recognition and machine learning is the ability to generalize to test data sampled from a distribution that is different from the training data distribution.
He notes that researchers in CfAR have been working on domain adaptation problems for more than a decade. With the new funding from Amazon, they also plan to investigate new weakly supervised methods for training networks, as well as advanced data augmentation algorithms based on adversarial learning.
—Story by Melissa Brachfeld