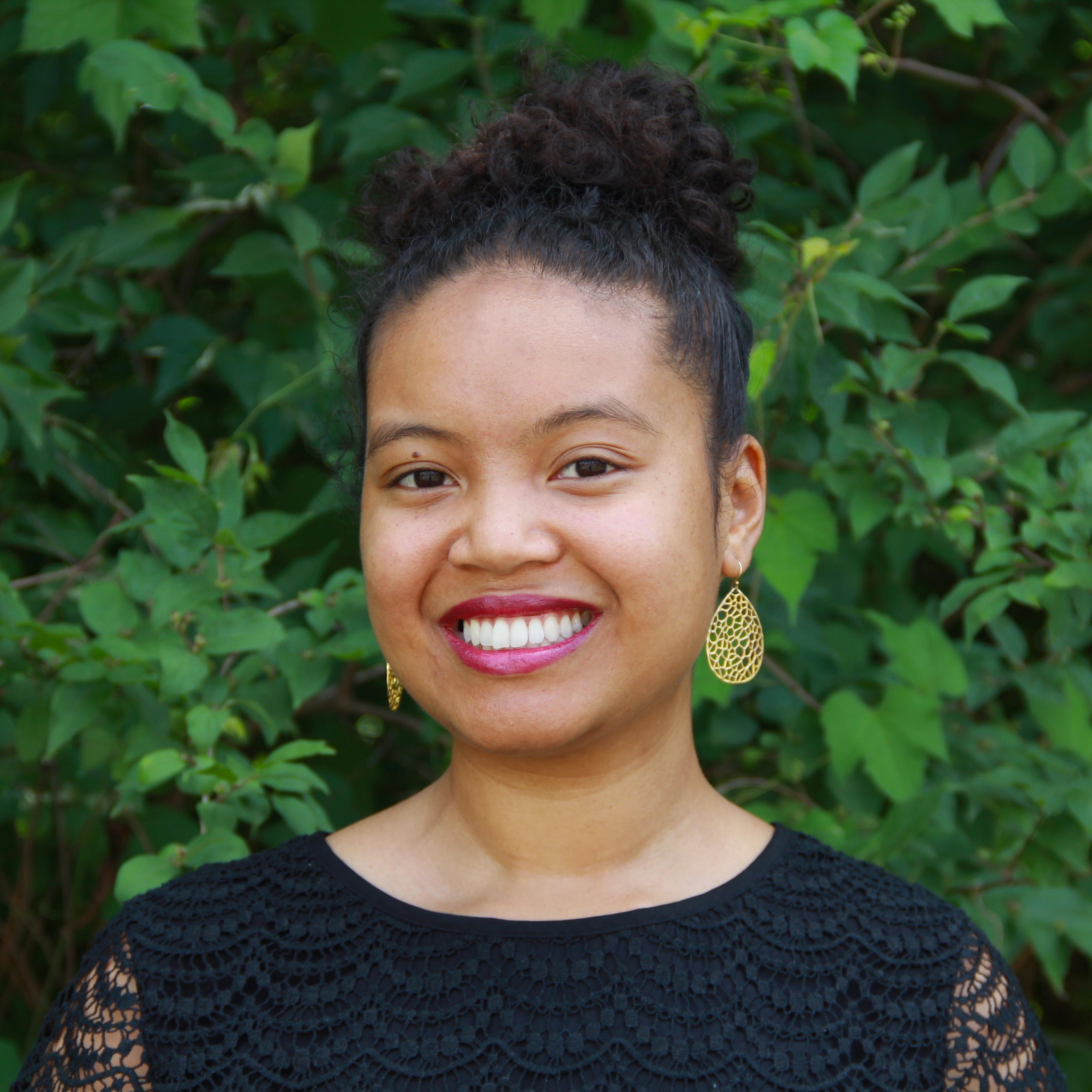
Leilani Battle, an assistant professor of computer science with an appointment in the University of Maryland Institute for Advanced Computer Studies, has received a National Science Foundation (NSF) grant to support the interactive exploration of massive datasets.
She is principal investigator of a Computer and Information Science and Engineering Research Initiation Initiative, which is expected to total $175,000 over two years.
The NSF award is designed to encourage immediate research independence after one receives their Ph.D. and starts their first academic position.
The funding supports Battle’s efforts to improve two key systems in exploratory data analysis tools: the visualization systems that provide graphical representations of the data, and the data management systems that efficiently manage large-scale data on the back end to support the analysis.
Scientists commonly use exploratory data analysis methods to gain insights from their data. However, increases in the number and granularity of data sources raise problems of scale that complicate the already difficult problem of developing tools that help analysts manage the often-changing goals and analysis trajectories suggested by their exploratory work.
Battle’s project, “Modeling Analysis Behavior to Support Interactive Exploration of Massive Datasets,” will produce dynamic optimization strategies for visual exploration systems, which infer the user's exploratory analysis goals over time, and deploy optimization algorithms tailored to the current analysis goal.
The development of these optimizations will be done in two phases. First, Battle will conduct a user study to characterize how users interact with visual exploration systems under different exploratory data analysis scenarios and system designs. Second, using the collected study data, a predictive query execution engine will be designed to infer users' analysis goals from log data and detect shifts in behaviors over time.
Battle's methods will address both human performance, or how effectively a user (a scientist or analyst) extracts insights and performs analysis tasks with a visual exploration system, and system performance, or how effectively the system responds to a user’s interactions. This research will enrich our understanding of how scientists and analysts explore data, Battle says, and will provide promising opportunities to improve coordination between humans and computers, ultimately supporting a more efficient data analysis process overall.