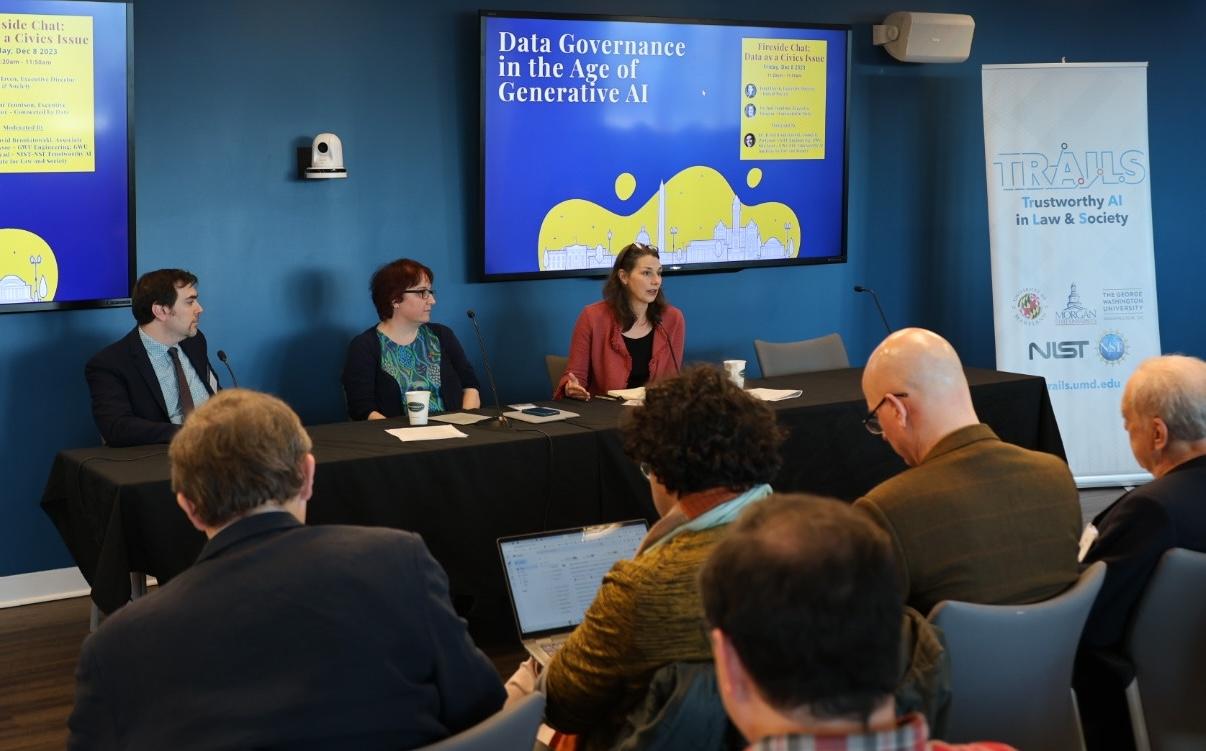
Millions of people around the world use generative AI chatbots such as ChatGPT to analyze information and solve complex problems. These bots are often built on large language models, which in turn are developed and trained on huge global datasets. Although these chatbots are awe-inspiring, they also often make mistakes or make up information. Hence, they could undermine longstanding democratic norms, as well as trust in information.
The Institute for Trustworthy AI in Law and Society (TRAILS) partnered with the Digital Trade and Data Governance Hub to host a conference to identify gaps in data governance related to large language models and discuss ideas to address them.
“Good data governance is a crucial aspect of any comprehensive attempt to govern AI,” says David Broniatowski, a TRAILS co-PI and associate professor of engineering management and systems engineering at George Washington University (GW) who moderated a panel on data as a civics issue. “At a time when significant attention is focused on regulating specific models and algorithms, this conference brings a much-needed focus to the data on which these algorithms and models operate.”
The December 7–8 conference brought experts from academia, industry, and government to the GW campus in Washington, D.C., to examine a wide range of issues including the current state of data governance; the role of open source vs. less open models, the long-term implications of these models; what governments are doing to govern generative AI, and new ideas for data governance. Some 130 people attended the conference in person, while 350 attended online.
The conference was the brainchild of Susan Ariel Aaronson, a co-PI at TRAILS and professor of international affairs at GW where she is also the founder and director of the Digital Trade and Data Governance Hub.
“There is no AI without data,” says Aaronson. “Large language models are built on proprietary and web-scraped data, but there is currently no way to ensure that these models are built on accurate, complete and representative datasets.”
Cody Buntain, a member of TRAILS and assistant professor of information studies at the University of Maryland (UMD), moderated a panel that examined this issue.
“How we collect data and where that data come from is often poorly considered or ignored when used to train models,” he explains. “These issues propagate when major, well-resourced organizations publish ‘foundation models’ that stakeholders can later fine-tune, and the data that trained the foundation models is poorly documented. Often, these issues are ignored for expediency.”
Buntain adds that the conference revealed how difficult problems like these are going to be to solve.
“It’s easy to identify problems, and stakeholders from many disciplines will identify unique ones. How we prioritize which problems to solve first or what tradeoffs are acceptable remain open questions,” he says.
In addition to Aaronson, Buntain and Broniatowski, the following TRAILS members also participated in the conference:
Hal Daumé III, Director of TRAILS and a professor of computer science at UMD, moderated a panel called “The Continuum of Closed and Open LLMs and their Implications for Data Governance.”
Tom Goldstein, a professor of computer science at UMD, TRAILS co-PI, and director of the UMD Center for Machine Learning, spoke as a panelist on the societal effects of data openness.
Daumé, Goldstein, and Buntain all have appointments in the University of Maryland Institute for Advanced Computer Studies, which provides technical and administrative support for TRAILS.